Companies in many industries, such as Life Sciences & Healthcare, stand on the cutting edge of an exciting transformation, harnessing Artificial Intelligence (AI) to accelerate innovation, streamline operations, and redefine customer experience and patient care. However, organizations now find themselves at a pivotal moment as a dramatic shift in the regulatory landscape under the Trump administration has plunged AI governance into uncharted waters. Companies that rely on AI face not only the immense promise of this technology but also unprecedented risks of litigation, compliance challenges, and ethical dilemmas.
AI compliance is no longer just a technical challenge; it is a multifaceted governance issue requiring collaboration across disciplines. Organizations must embrace a proactive, multidisciplinary approach to AI risk management, ensuring regulatory compliance, ethical AI use, and operational transparency. To effectively manage regulatory changes and the needs of the business, proactive governance, clear accountability, consistent monitoring, and defensible approaches are needed.
Recognizing that legal exposure in the AI realm can stem not only from adverse outcomes but also from a lack of explainability or human oversight, we recommend that organizations using AI in any form create robust governance structures, develop transparency protocols, and subject their AI tools to rigorous validation and bias audits.
The Regulatory Storm
Consider January 2025, when two significant Executive Orders changed the rules overnight. First, the Executive Order “Ending Illegal Discrimination and Restoring Merit-Based Opportunity”[1] ended the long-standing affirmative action mandates for federal contractors while imposing new certification requirements on them. This shift created a legal minefield, heightening risks under the False Claims Act. Though non-discrimination laws remain in effect, employers must now navigate complex legal waters without clear federal guidance, making them vulnerable to costly litigation and whistleblower claims.
Days later, another Trump Executive Order, “Removing Barriers to American Leadership in AI,”[2] revoked Biden’s AI policy, “Safe, Secure, and Trustworthy AI.”[3] The new Executive Order emphasizes deregulation and innovation but leaves companies largely on their own to manage compliance, transparency, and ethical standards.
While the FDA’s draft guidelines on AI use in drug development[4] and AI-enabled medical devices,[5] as well as the NIST’s AI Risk Management Framework[6] are still in place , the extent to which these guidelines will influence enforcement actions remains uncertain. This regulatory ambiguity places an even greater responsibility on individual organizations to establish self-directed AI governance programs that are defensible.
Caught Between Innovation and Compliance
The convergence of AI innovation and regulatory uncertainty places organizations in a delicate position. While companies are eager to harness AI’s potential not all company personnel may be aware of the growing compliance and litigation risks involved. Two areas where this tension is most pronounced are the life sciences/healthcare and the employment function. In both of these areas, AI tools promise to improve efficiency and outcomes but simultaneously present serious legal, ethical, and operational risks. In life sciences/healthcare, companies are using AI to process healthcare claims, as well as assist in the diagnosis of results captured by imaging machines. And across a range of industries, AI is being used to screen candidate applications for positions, as well as to evaluate travel and entertainment expenses submitted by employees for reimbursement. Too often, these uses of AI are applied without proper regard for their potential litigation, regulatory compliance, and governance risks.
Litigation risk is growing with cases already being filed. Among these are Kisting-Leung v. Cigna[7] and Estate of Lokken v. UnitedHealth,[8] where the plaintiffs allege that insurers used AI systems to automate claim denials without individualized medical review. Lawsuits like these argue that such practices may violate good faith obligations, state insurance regulations, and consumer protection laws, particularly when patients are denied necessary care based on opaque or allegedly biased AI-driven recommendations. In addition to any Federal laws or guidance, companies need to consider State laws and guidance, such as California’s SB1120,[9] which mandates human oversight in AI-driven healthcare decisions and requires fairness principles in utilization review processes.
In Virginia, Governor Glenn Youngkin’s recent veto of the “High-Risk Artificial Intelligence Developer and Deployer Act”[10] illustrates the growing complexity of AI regulation at the state level. Although the bill aimed to curb algorithmic discrimination by requiring documentation of AI systems’ intended use, risks, and performance, and mandating risk management and impact assessments, it drew criticism for potentially imposing administrative burdens without clear implementation guidance. The mixed reaction not only reflects broader uncertainty around how best to regulate AI but also reinforces the need for companies to proactively monitor and prepare for emerging state and federal frameworks.
While AI’s expanding role in life sciences clinical trials introduces the opportunity to improve health outcomes, it also introduces additional compliance risks. For example, AI systems are increasingly used to optimize trial design, match patients, and analyze safety data in real-time. However, these innovations need to comply with FDA guidance on model validation, Good Clinical Practice (GCP) standards, and bias mitigation. Failure to ensure scientific reliability or demographic fairness could undermine trial integrity and violate regulatory guidance. For instance, AI models trained on historically homogeneous datasets could risk excluding underrepresented populations, potentially skewing results, not meeting certain endpoints, or potentially misrepresenting effectiveness across demographic populations.
In the employment context, AI introduces the possibility of more efficient identification of qualified candidates but also increases potential regulatory and litigation risks if the tools are not adequately evaluated for bias. The optimal way of handling these risks is further clouded given the rollback of Biden-era AI guidance, the removal of EEOC and DOL resources, and the lack of federal replacement guidance going forward. But it is critical to remember that anti-discrimination laws, including Title VII and the Americans with Disabilities Act (ADA), remain fully in force. Moreover, local and state-level regulations like the Illinois AI Hiring Act[11] and New York City’s Local Law 144[12] are filling the federal void. And with or without Federal or State guidance, employers failing to validate their tools or provide notice to candidates may face litigation and reputational damage.
A common thread balancing the AI opportunities with their associated risks across any domain is the need for defensible governance. We recommend that organizations subject their AI tools to rigorous validation, bias audits, and transparency protocols. Legal exposure can stem not only from adverse outcomes but also from a lack of explainability or human oversight. Regulatory bodies like the FTC, FDA, and HHS signaled increasing scrutiny just before the Trump administration took the helm. Whether and how they will change course remains to be seen. However, state laws and industry codes of conduct, such as those emerging from the Coalition for Health AI or the ONC’s Predictive Decision Support Framework, are reshaping the compliance landscape.[13] In addition, for AI tools provided by third parties, we suggest that companies obtain a clear understanding of responsibility and liability involving the validation of the tool’s decision making accuracy, as well as which party is responsible for that accuracy. Relatedly, it is important to understand whether limitation on liability and indemnification provisions sufficiently cover enterprise risk in the event of a regulatory or litigation issue.
In this environment, AI adoption without governance is a gamble that need not – and should not – be taken. Organizations can bridge the gap between innovation and compliance by embedding ethics, transparency, and legal review into their AI strategies and implementation. Doing so is not just a regulatory imperative, it is essential to preserving trust, equity, and safety in the rapidly evolving AI frontier.
Navigating the Storm: Strategic Decisions for AI Governance
To survive and thrive amid this uncertainty, companies must make critical governance decisions. These decisions are not mere formalities—they are essential to preserving organizational integrity, patient safety, and employee fairness:
1. Establish an AI Governance Program
A well-defined AI governance program should consider the following actions:
- Form an AI governance steering committee to ensure alignment with evolving regulations and ethical standards while achieving business goals.
- Engage a multidisciplinary team, including data scientists, human resources, regulatory and compliance experts, under the advice of legal counsel, to oversee AI system design, deployment, and monitoring.
- Adopt an AI risk management framework that aligns with NIST AI standards and industry best practices.
- Focus on ensuring transparency and accountability in AI-driven decision-making.
- Consider having Legal, Procurement, and IT create a model for engaging with third party providers of AI tools.
2. Conduct Comprehensive Bias Audits and Model Validation
Bias and fairness must be actively monitored, particularly in hiring algorithms, medical AI diagnostics, and claims processing tools. Leading practices include:
- Regular AI bias audits to assess disparate impacts across demographic groups.
- Validating AI predictions and generative outputs to ensure fairness, accuracy, and regulatory compliance.
- Cross-disciplinary review of AI performance, involving statisticians, ethicists, legal experts, and end-users to assess unintended biases or discriminatory patterns.
- Focus on properly documenting the tests performed and results obtained over the iterations of development and use.
3. Strengthen Transparency and Explainability
AI-driven decisions must be explainable, understandable, and defensible when engaging with regulators, stakeholders, and affected individuals. Organizations should consider:
- Maintaining clear documentation on how AI systems function, make decisions, and generate outputs.
- Collaborating consistently across disciplines, integrating perspectives from software engineers, compliance officers, HR professionals, and medical practitioners to improve AI interpretability.
- Focusing on regulatory compliance with AI transparency requirements at global, federal, and state levels.
4. Proactively Address Liability and Ethical Risks
Organizations using AI should consider assessing liability exposure and implementing ethical safeguards. This could include:
- Legal review processes to determine liability in AI-driven employment and healthcare decisions.
- Procurement and legal protocols regarding engaging with third parties and consideration of fourth parties, including controls around ownership of liability and indemnification.
- AI ethics committees to oversee high-risk AI applications.
- State, federal, and global legal compliance strategies, ensuring adherence to Title VII, ADA, HIPAA, GDPR, and emerging AI-specific regulations.
- Input from all stakeholders, including patient advocacy groups, employee representatives, and public policy experts, to align AI decisions with ethical and legal standards.
- Auditing third parties, and if possible, fourth parties on a consistent basis to evaluate algorithms and tools they are providing, as well as their management of your data.
5. Invest in AI Literacy Across the Organization
AI literacy is critical for HR teams, healthcare providers, compliance officers, and employees interacting with AI-driven decisions. Organizations should:
- Provide AI training and education to non-technical stakeholders to ensure informed oversight.
- Host cross-functional AI workshops to bring together legal, technical, and ethical perspectives.
- Encourage continuous learning on AI governance, bias mitigation, and compliance risks.
Seizing the Opportunity: A Call to Action
The AI regulatory landscape is undergoing significant transformation. While recent executive orders have rolled back certain AI governance requirements, they do not eliminate fundamental compliance obligations under federal laws such as Title VII, ADA, and the False Claims Act. This shift changes the risk calculus, making it even more critical for organizations to establish strong internal governance structures to mitigate compliance risks and ensure responsible AI use.
AI compliance is not just a technical challenge but also it is a multifaceted governance issue requiring collaboration across disciplines. Organizations must embrace a proactive, multidisciplinary approach to AI risk management. By integrating legal, regulatory, compliance, ethical, and technical expertise, businesses can navigate the AI landscape that is evolving at a dizzying pace while mitigating risks and unlocking AI’s full potential responsibly.
To navigate this dynamic environment, businesses should take an informed, proactive approach, ensuring that AI initiatives are compliant, ethical, and strategically aligned with both current laws and future regulatory trends. The establishment of a centralized AI steering committee is a strategic necessity to manage AI-related risks and compliance challenges effectively. Based on our experience to date, Resolution Economics is well-positioned to assist organizations in developing multidisciplinary AI governance frameworks, implementing and testing AI algorithms, and executing the associated controls. Our firm provides advisory, investigative, data analytics, and litigation support across the areas of Labor and Employment, Life Sciences and Healthcare, Financial Advisory, AI, and HR. We are here to help.
Contact us to learn more:
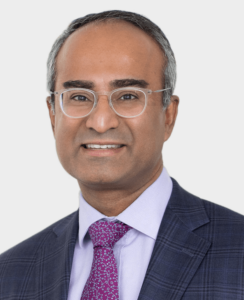
Partner
ybahl@resecon.com
646.424.4330
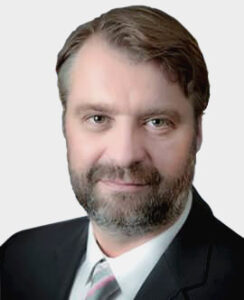
Director
gurkan@resecon.com
202.800.2723

Margo Pave
Director
mpave@resecon.com
202.524.1658
[1] https://www.whitehouse.gov/presidential-actions/2025/01/ending-illegal-discrimination-and-restoring-merit-based-opportunity/
[2] https://www.whitehouse.gov/presidential-actions/2025/01/removing-barriers-to-american-leadership-in-artificial-intelligence/
[3] https://www.federalregister.gov/documents/2023/11/01/2023-24283/safe-secure-and-trustworthy-development-and-use-of-artificial-intelligence
[4] “Considerations for the Use of Artificial Intelligence to Support Regulatory Decision-Making for Drug and Biological Products – Guidance for Industry and Other Interested Parties – Draft Guidance,” January 2025, https://www.fda.gov/media/184830/download
[5] “Artificial Intelligence-Enabled Device Software Functions: Lifecycle Management and Marketing Submission Recommendations – Draft Guidance for Industry and Food and Drug Administration Staff,” January 7, 2025, https://www.fda.gov/media/184856/download
[6] https://www.nist.gov/itl/ai-risk-management-framework
[7] Consumers in California filed a class-action lawsuit against national health insurer Cigna Corporation, alleging that its algorithm-driven denial of medical claims constituted breach of the implied covenant of good faith and fair dealing, unjust enrichment, intentional interference with contractual relations, and violations of California’s Unfair Competition Law. These denials potentially exposed patients to significant out-of-pocket expenses or forced them to forego essential medical care. The case underscores broader concerns regarding the risks posed by insurers’ reliance on AI-driven decision-making, particularly its potential impact on patient access to necessary healthcare (https://litigationtracker.law.georgetown.edu/litigation/kisting-leung-et-al-v-cigna-corporation-et-al/).
[8] Plaintiffs representing patients with terminated post-acute care coverage allege that a national health insurer’s use of AI to deny Medicare Advantage claims constitutes breach of contract, breach of the implied covenant of good faith and fair dealing, unjust enrichment, and insurance bad faith. Relying on AI over clinical provider assessments can deny patients essential treatments and harm their health. Wrongful claim denials by AI-reliant health insurers could jeopardize access to vital health care services (https://litigationtracker.law.georgetown.edu/litigation/estate-of-gene-b-lokken-the-et-al-v-unitedhealth-group-inc-et-al/).
[9] SB 1120 went into effect in January 2025. “This bill would require a health care service plan or disability insurer, including a specialized health care service plan or specialized health insurer, that uses an artificial intelligence, algorithm, or other software tool for the purpose of utilization review or utilization management functions, or that contracts with or otherwise works through an entity that uses that type of tool, to ensure compliance with specified requirements, including that the artificial intelligence, algorithm, or other software tool bases its determination on specified information and is fairly and equitably applied, as specified. Because a willful violation of these provisions by a health care service plan would be a crime, this bill would impose a state-mandated local program.” https://legiscan.com/CA/text/SB1120/id/3023335#:~:text=This%20bill%20would%20require%20a,management%20functions%2C%20or%20that%20contracts
[10] https://lis.virginia.gov/bill-details/20251/HB2094/text/HB2094
[11] https://ilga.gov/legislation/BillStatus.asp?GA=103&SessionID=112&DocTypeID=HB&DocNum=3773
[12] https://legistar.council.nyc.gov/LegislationDetail.aspx?ID=4344524&GUID=B051915D-A9AC-451E-81F8-6596032FA3F9&Options=ID%7CText%7C&Search=
[13] “Health Care’s AI Transformation: Managing Risks and Rewards in an Evolving Landscape” by Lisa Amanti, Katherine Snow, and Alya Sulaiman, ABA The SciTech Lawyer, Winter 2025, Vol 21, No 2